Abstract: Representing 3D objects and scenes with neural radiance fields has become very popular over the last years. Recently, surface-based representations have been proposed, that allow to reconstruct 3D objects from simple photographs.
However, most current techniques require an accurate camera calibration, i.e. camera parameters corresponding to each image, which is often a difficult task to do in real-life situations. To this end, we propose a method for learning 3D surfaces from noisy camera parameters. We show that we can learn camera parameters together with learning the surface representation, and demonstrate good quality 3D surface reconstruction even with noisy camera observations.
Click here to view our AAAI-23 poster
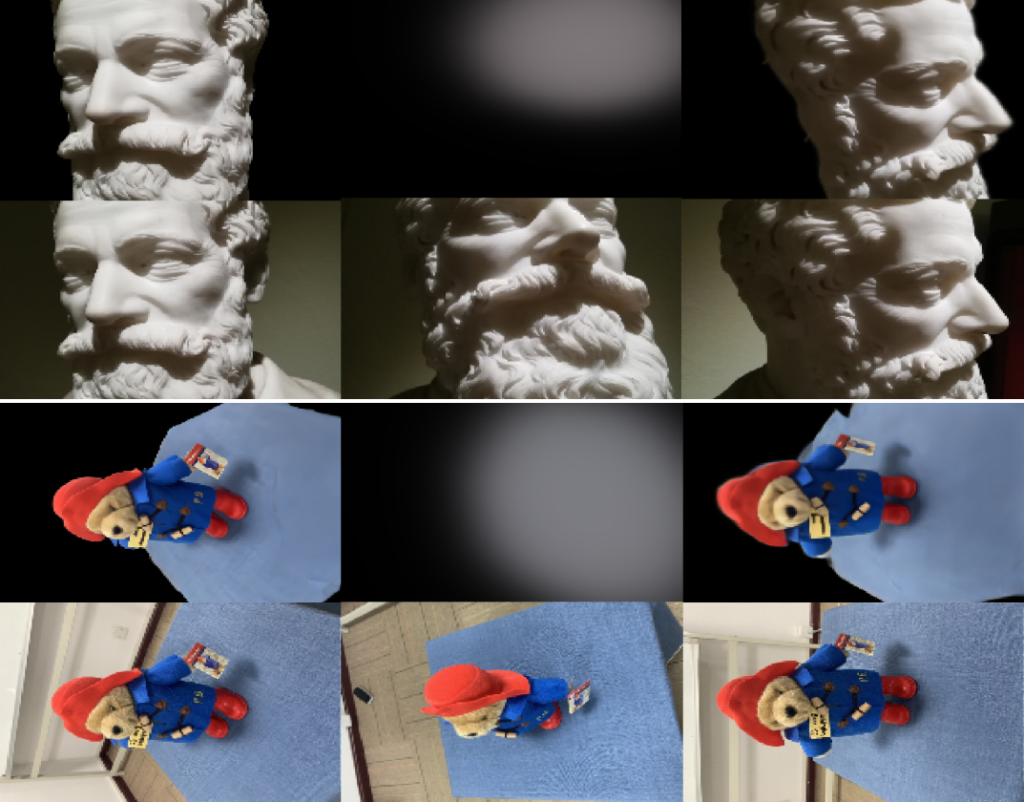
This work was published in the AAAI-23 Student Abstract and Poster Program: Sarthak Gupta, Patrik Huber, Neural Implicit Surface Reconstruction from Noisy Camera Observations, AAAI Student Abstract, AAAI 2023, Washington DC, USA.
Paper (with supplementary material): https://arxiv.org/abs/2210.01548